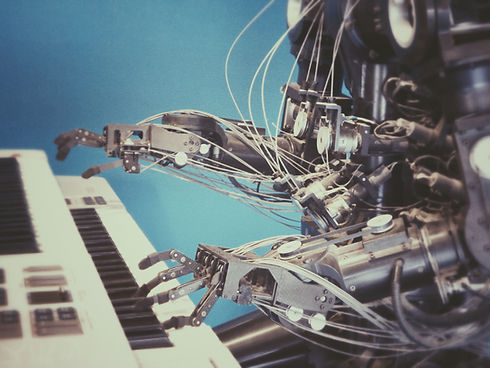
BLOCK 3: LEARNING FROM MACHINES
Big data is facilitating radical new approaches to understanding and delivering learning. What new regimes of educational theory and practice are being instantiated by these technical development in data mining, algorithmic thinking, AI, machine learning and predictive analytics?
What Are the Real Dangers of Artificial Intelligence?
Adam Greenfield speaks about some of the more human implications of artificial intelligence: "The really troubling implication of artificial intelligence for the world of work is this: it will not be limited to automating away physical labour."
What is Machine Learning?
Put simply, machine learning is a subset of artificial intelligence which involves the application of algorithms and mathematical and statistical models to big data sets by programming computer systems to perform specific tasks without explicit instructions, but rather by relying on the computational analysis of patterns to draw inferences and make predictions. There are three basic types of machine learning:
SUPERVISED LEARNING
Supervised learning algorithms build a mathematical model of a given set of data that contains both the inputs and the desired outputs. This covers a range of different task-driven models used for classification and regression, the latter often used for forecasting and prediction.
UNSUPERVISED LEARNING
Unsupervised learning algorithms take a set of data that contains only inputs, and find structure in the data, like grouping or clustering of data points. This covers models used for clustering and dimensionality reduction, such as recommender systems and text mining.
REINFORCEMENT LEARNING
Reinforcement learning exposes the algorithms to to an environment where it trains itself using trial and error, continually learning from past experience to capture knowledge and make better decisions. Such models are capable of tasks like real-time decision-making and robot navigation.
Machine Learning, Predictive Analytics and the Personalisation of Education
Machine learning is concerned with analysing data to classify and predict events or identify relationships among people, things, and processes. A vast proliferation of techniques, methods and practices derived from developments primarily in mathematics, statistics and computer science, machine learning works to transform or construct “some kind of shape on the data and using that shape to discover, decide, classify, rank, cluster, recommend, label or predict what is happening or what will happen” (Mackenzie, 2015, p. 432). One of the key functions of machine learning is prediction: “machine learning has produced ‘learning algorithms’—or just ‘learners’—that can process vast databases of historic and real-time data in order to generate predictions and probabilities” (2017, 7-8). Predictive modelling techniques such as logistic regression models, the naive Bayes classifier, k-nearest neighbours, decision trees and neural networks are increasingly used to ‘personalise’ learning, predicting behaviour, adapting and responding, and learning based on new inputs provided by learners. The authority, agency and power being entrusted to such algorithms is immense.
Characterised as the “the re-emergence of empiricism”, datafication, learning analytics, machine learning have promoted a radical new behaviourism in education (Knox et al., 2019), a type of reinforcement learning now underpinning “many of the psychological techniques used by data scientists and social media designers for online ‘behaviour modification’ and ‘behaviour engineering’” (Knox et al., 2019, p. 5). For these new data-driven behaviourist technologies, “the task is not just to discern learning through observation of behaviour, but to actively modify and determine it through direct and intentional control of the environment” (p. 5-6). Such approaches to learning, with positive and negative reinforcement, with learners “increasingly ‘nudged’ towards predefined modes of participation and behaviour” (p. 11-12)”, are really outdated, totally out-of-line with contemporary understandings of learning. Yet the lure persists, because the unreliable objectivity of big data, the notion that enough with data we might find an answer, some transcendental truth about learning, eclipses any substantive critique.
READING
Watters A. (2017, December 23) Education technology and the new behaviourism, Hack Education [blog], http://hackeducation.com/2017/12/23/top-ed-tech-trends-social-emotional-learning
Offering a critique of B. F. Skinner's behaviourist learning theories, this blog post by Audrey Watters examines trends in neurotechnologies and neuroeducation, suggesting that these are inadvertently reinforcing a new behaviourism in educational policy, theory and practice under the guise of 'behaviour design'. Consider: What are the underlying pedagogical assumptions baked into the educational technologies you use in your teaching?
“[F]uture education may tend toward very specific forms of behavioural governance – a ‘machine behaviourism’ – entailing potent combinations of radical behaviourist theories and powerful machine learning systems, that appear to work against notions of student autonomy and participation, seeking to intervene in educational conduct and shape learner behaviour towards predefined aims.”
Jeremy Knox, Ben Williamson & Sian Bayne, 'Machine behaviourism' (2019)
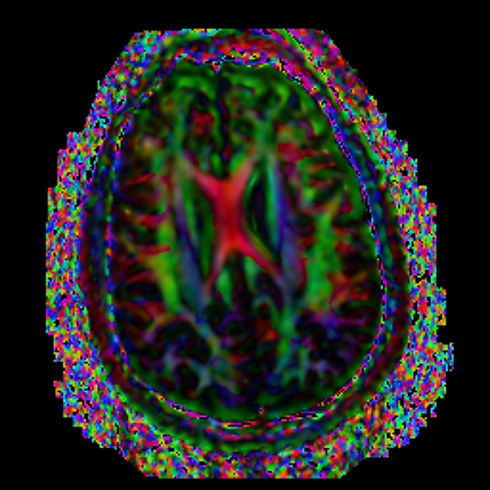
What are Individuality, Difference and Diversity to a Machine?
The capacity of machine learning to classify depends on its learning to “recognise the differences between categories that themselves remain fixed”, and many of which “start from a data sample that has already been classified or labelled by someone” (Mackenzie, 2015, p. 433). Machine learning and predictive analytics depend on classification, which “presumes the existences of classes, and attributes that define membership of classes” (p. 433). Even more dangerously, big data, automation, algorithms and machine learning result in the datafied construction of categories of identity—the creation of a new “algorithmic identify” (Cheney-Lippold, 2011), producing a picture of the learner based on available data, pre-programmed rules, pre-determined categories and classifications. But classification, categorisation, generalisation, vectorisation, optimisation, approximation all tend towards the general rather than the particular, flattening individuality, difference and diversity. And if differences are not captured by the data, do they exist at all? Pedagogical practice, educational policy and institution strategy proceed on the basis of the data collected, threatening to lose of leave behind that will falls through the cracks.
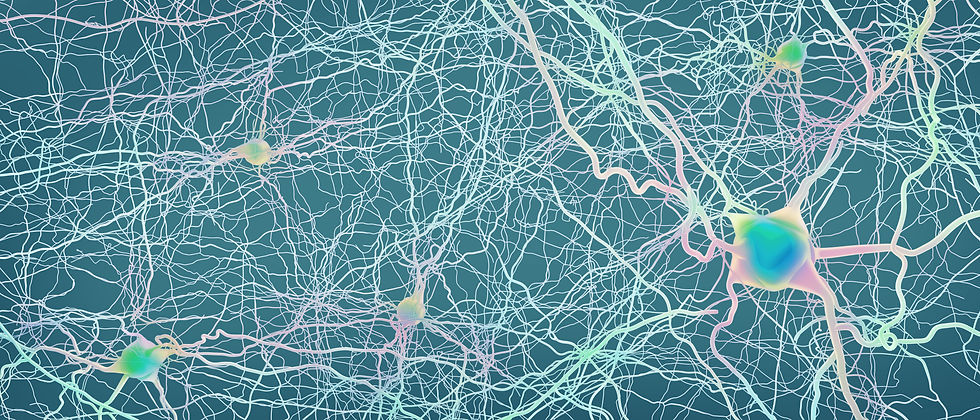
Neuro-Biological Determinism in the Learning Sciences
Datafication has significant ramifications for education beyond the influence of big data and machine learning. Advances in educational neuroscience, as well as the development of brain-based learning and social-emotional learning, are leaning on the techno-scientific objectivity and validity afforded big data to articulate a science of learning. But through this mechanisation of the brain, neuroscience must confront “significant questions about how the human brain may be examined, modelled, understood and made amenable to manipulation and modification in years and decades to come” (Williamson, 2019, p. 66). We risk reducing the human brain to a series of data-points, the learning brain, indeed the learner, “rendered reductively as algorithmic or computational processes, which are therefore amenable to being optimised by the application of cognitive computing algorithms” (2017b, p. 91). Such an approach “treats reductive forms of neuroscientific evidence about the neurobiological processes of young people as the target for strategic intervention” (p. 92). As neuroscience continues to detect, characterise and classify behavioural patterns, “with objectivity ascribed to the datafication of students’ psychological traits, characteristics and attributes” (Williamson & Piattoeva, 2019, p. 66), it is contributing to a radical “reimagining of the human subject—one understood algorithmically in terms of computable cognition and programmable brain functions” (Williamson, 2017b, p. 82). A danger here is that “complex human and social activities—and the values and assumptions held about them—are operationalised by being translated into a functional interaction of models, goals, data, variables, indicators, and outcomes” (p. 84), the human, the learner, reduced to an over-determined set of data-points.
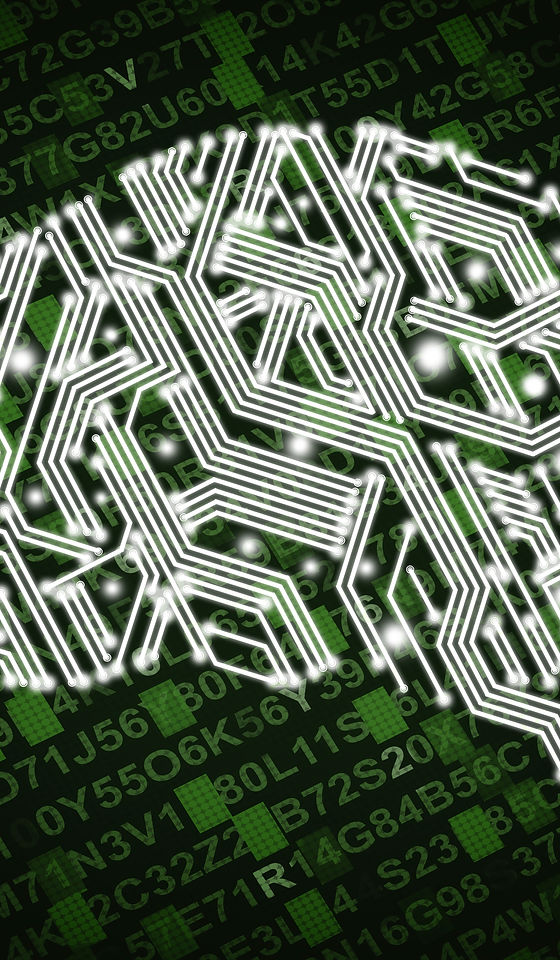
ACTIVITY 3: ANALYSIS
Big Data, the Learning Sciences and Behavioural Governance
To conclude: How is the learner being made amenable to datafication? What examples can you find in your of educational setting? How does the data-driven intervention into education of algorithmic thinking, artificial intelligence, automated decision-making, machine learning techniques, and predictive analytics, change the way we understand and approach learners and learning?
Record your thoughts, in 200-300 words, and share your response to the Padlet below. Feel free to comment on and critique each other's work.