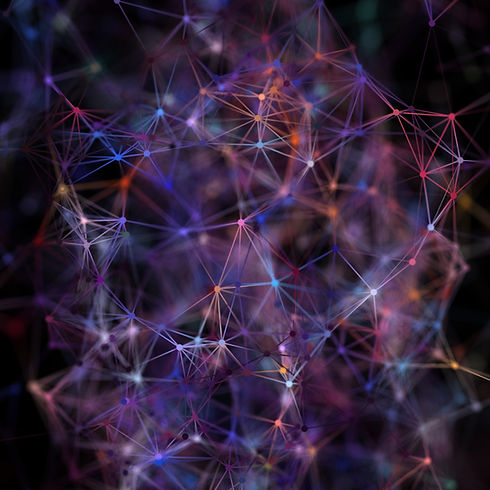
BLOCK 2: RAW DATA IS AN OXYMORON
Data is not neutral, but always already manipulated, from the moment of conception, even before collection. Data is always already interpreted, imagined, subjective.
The Unreliable Objectivity of Big Data
Perhaps the greatest unintended consequence of datafication is the displacement of the quality of evidence by the sheer quantity of data. This is the ‘mythology’ of big data, “the widespread belief that large data sets offer a higher form of intelligence and knowledge that can generate insights that were previously impossible, with the aura of truth, objectivity, and accuracy” (boyd & Crawford, 2012, p. 663). Through sheer volume of data and analysis, educational research hopes to be rendered objective, quantifiable and apolitical., afforded scientific vali Big data and quantification become “allied with objectivity” not for their actual accuracy, but because these measures of education “promise a new era of accuracy and objectivity in scientifically-informed educational policymaking” (Williamson & Piattoeva, 2019, p. 64). We are apparently capturing learners and learning with ever-increasing objective fidelity. However, “the objectivity of a data-scientific form of education policy is in fact a precarious achievement”, itself produced through “processes that involve networks of actors, technologies, policy activity, and scientific expertise” (p. 64). The consequence is that our supposedly objective data-points, already proxy for actual learning, overshadow other forms of evidence due to the uncritical weight placed on an unreliable data-scientific objectivity.
Despite the ubiquity of the phrase raw data — over seventeen million hits on Google as of this writing — we think a few moments of reflection will be enough to see its self-contradiction, to see... that data are always already “cooked” and never entirely “raw.”
Lisa Gitelman and Virginia Jackson, "Raw Data" is an Oxymoron (2013)
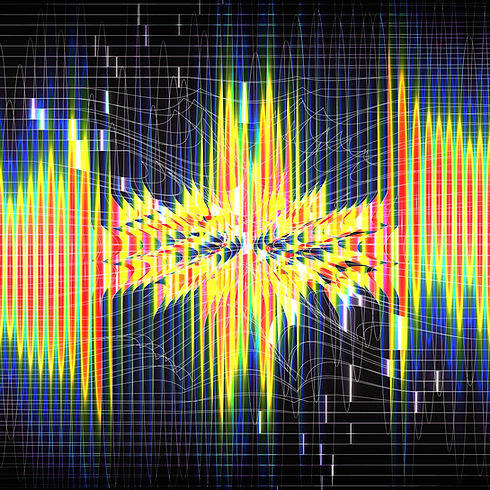
“The rise of digital data analytics has catalysed attempts to render ever-more ‘objective’ measures of education. New forms of data-driven analysis performed through education technologies appear to promise a new era of accuracy and objectivity in scientifically-informed educational policymaking. The production of data about students represent attempts to capture students in increasingly objective fidelity. In so doing, these scientifically-produced measures may become increasingly influential in directing policymakers toward problems for intervention, as they produce ‘policy-relevant knowledge’ with the ‘objectivity,’ neutrality and impartiality ascribed to the authority of pure science itself. While objectivity is at the centre of scientific modes of data-driven ‘evidence-based policy’, however, objectivity is itself produced through processes that involve networks of actors, technologies, policy activity, and scientific expertise – revealing how the objectivity of a data-scientific form of education policy is in fact a precarious achievement.”
Ben Williamson & Nelli Piattoeva, 'Objectivity as standardization in data-scientific education policy, technology and governance' (2019)
Stop Assuming Data, Algorithms and AI Are Objective
In this TED Talk, Mata Haggis-Burridge discusses how the big data that is collected to build AI systems are merely a reflection of past patterns. In this way, despite the best intentions of developers, discrimination, racism and prejudice sneak into the systems we now rely on to eliminate such human biases. In order to break with the patterns of our past, he argues that we need to recognise their flaws and teach the algorithms of the future to do the same.
Big Data, Algorithmic Thinking and Automated Decision-Making
Big data undoubtedly represents a massive paradigm shift in the way we understand and think about education. It is spoken about in terms of enhancing efficiency, increasing transparency, supporting competitiveness and evaluating the performance of learners and teachers—all desirable objectives for a marketised education sector, with both intended and unintended consequences of datafication that need to be interrogated.
Big data raises obvious ethical questions around informed consent, privacy and surveillance, as well as wider questions about what types of data should be collected, combined and analysed, and the purposes to which this should be put. There are also concerns about accountability, autonomy, agency, control, equity, bias, fairness and social justice.
An algorithm is only as intelligent as the human programming it, and data only as reliable as the agent collecting, sorting, analysing and interpreting it; hence an unintended consequences of datafication turns out to be creeping racism, sexism, and other forms of gender-based discrimination. Data and algorithms encode social bias, and need to be challenged and critiqued at every level of operation.
Facilitated by big data and datafication, the growth of automated or algorithmic decision-making by governments, private corporations and educational institutions subjects information, knowledge and discourse to the “procedural logics that undergird all computation”, necessarily shaping human behaviour, indeed shaping how we perceive ourselves and our society. Algorithmic processes take on increasing weight and responsibility, assume greater agency and control; indeed, “authority is increasingly expressed algorithmically” (Pasquale, 2015, p. 8), even as the “values and prerogatives that the encoded rules enact are hidden within black boxes” (p. 1).
What choices and decisions are being pre-programmed into education? Who is being trusted to program the algorithm? What are their values and beliefs about education? As we embed computational and algorithmic thinking into education, we need to understand not just how algorithms make choices, but how they collect and compute information that informs and shapes choice.
READING
Shivhare, N. (2018, March 8), AI in schools — here’s what we need to consider, The Conversation, https://theconversation.com/ai-in-schools-heres-what-we-need-to-consider-109450
In this article from the Conversation, Neha Shivhare surveys some of the issues arising from the adoption of artificial intelligence, particularly tutoring systems, in education. She suggests that perhaps "need to confront ambiguous questions raised by the reality that teaching-learning interaction can now occur without the personal mediation of a teacher." What do you think? Can learning occur without the personal mediation of the teacher?
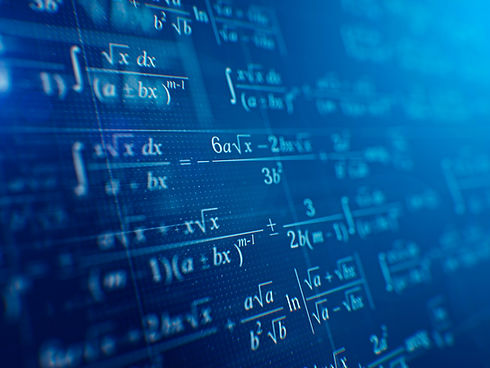
AIDA BY PEARSON
Can Robots Replace Teachers?
Here's a recent case study in personalised learning: Consider Aida, the artificially intelligent calculus tutor, developed and launched in November 2019 by Pearson. Begin by watching this promo video:
You can learn more about Aida by visiting the Pearson website, and for some broader context, Aida was featured in a few of the higher education blogs:
Friedman, S. (2019, November 12) Pearson Calculus App Uses AI to Provide Personalized Learning. Campus Technology.
McKenzie, L. (2019, November 18) Pearson Launches Calculus Homework Helper. Inside Higher Ed.
Aida claims to be able to pinpoint learners' mistakes without teacher intervention, providing instant feedback on work, helping learners understand where they went wrong, adapting to their strengths and weaknesses, and customising future study suggestions.
But what potential biases and prejudices are hidden within Aida's algorithms and programming? How does a tool such as Aida understand learners and learning? What types of learning behaviour does the tool encourage or favour? How does it shape or reshape what learning is?

ACTIVITY 2:
CASE STUDY
Does More Data Actually Allow Us To See Better?
Let's pause to think about algorithmic bias. Consider the technologies used for learning in your own educational setting. Select one from the list below. What potential biases may be encoded into these technologies? What types of learning behaviour do these tools encourage or favour?
Adaptive/Personalised Learning
Eye-Tracking
Facial Recognition
Learning Analytics
Mobile Learning
Share your case study on the Padlet below. Feel free to comment on each other's responses.