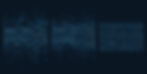
HUMANISING (BIG) DATA
Datafication, the Data-Scientific Turn and the Future of Education
What is the future of education in the wake of mass datafication? How is big data changing our understanding of learners and learning? This open educational resource examines the techno-scientific culture of measurement that is being advanced through the mass datafication of education, in all its mess and complexity. How are such technical developments as algorithmic thinking, artificial intelligence, data mining, machine learning and predictive analytics, reshaping pedagogical practice, educational policy, and institutional strategy?
Surveying developments such as the emergence of artificial intelligence, algorithmic thinking and automated decision-making into education, the learning theories and governance regimes instantiated by machine learning techniques and predictive analytics, and the neuro-biological determinism lurking beneath the contemporary learning sciences, this OER hopes to demonstrate that, if unchecked, the sheer volume and velocity of big data—for all the good it can do and is doing to aid our understanding of learning—also threatens to reproduce an outdated determinism which runs counter to contemporary thinking about how learners learn.
This OER consists of three sections, each with its own capstone activity asking you to reflect critically and analyse your own encounters with and uses of big data, artificial intelligence and machine learning techniques in educational settings:
DATA AND ITS DISCONTENTS
Datafication and its Discontents explains the rise and lure of big data, and considers its (unintended) consequences for education and society.
RAW DATA IS AN OXYMORON
Raw Data is an Oxymoron begins to examine the the effects of datafication and quantification on how we understand learners and learning.
LEARNING FROM MACHINES
Learning from Machines critiques new regimes of educational theory and practice instantiated by data mining, algorithmic thinking, AI and machine learning.
.jpeg)
Learning Objectives
By completing this OER, you will learn to:
Describe the effects of big data and datafication on education and society.
Critically evaluate the use of algorithms, artificial intelligence and machine learning in educational settings.
Analyse how the techno-scientific datafication and quantification of education changes our understanding of learners and learning.
.jpeg)
Before You Start
These are the tools you will need to get the most out of this OER:
Three hours. Roughly one hour per block. That's all it should take to work through this resource.
Internet access. All of the essential resources used here are freely available online.
A notebook. Or some other equipment for jotting down your ideas. Pen and some paper will work.
An enquiring mind. And a critical mindset. The ability to challenge and be challenged.
Ready? Let's Go!
Select Block 1 below to get started.
“The world is one big data problem. There’s a bit of arrogance in that, and a bit of truth as well.”